At present we just now beginning to see AI techniques being offered as commercial products to reduce imaging time and improve image quality. Deep learning (DL) methods can be applied at one or more points along the imaging pipeline, including data acquisition and processing, channel combination, image generation, and post-processing. We will begin our discussion at the end of the pipeline (post-processing), where post-processing DL methods are used to enhance MR images only after they have been fully reconstructed by the scanner.
DL-based Post-processing
These methods are the easiest to implement and most widely used. Also known as "DICOM-AI", DL-based post-processing operates in "image space" and makes use of a wide range of techniques already developed for photography, video, and non-medical imaging. Since it do not rely on proprietary knowledge of acquisition or reconstruction techniques, DL-based post-processing can be applied to images from any MR vendor. Current applications include noise reduction, improved and super-resolution, reduction of gadolinium dose, metal artifact reduction, motion artifact reduction, synthetic image production, and image registration.
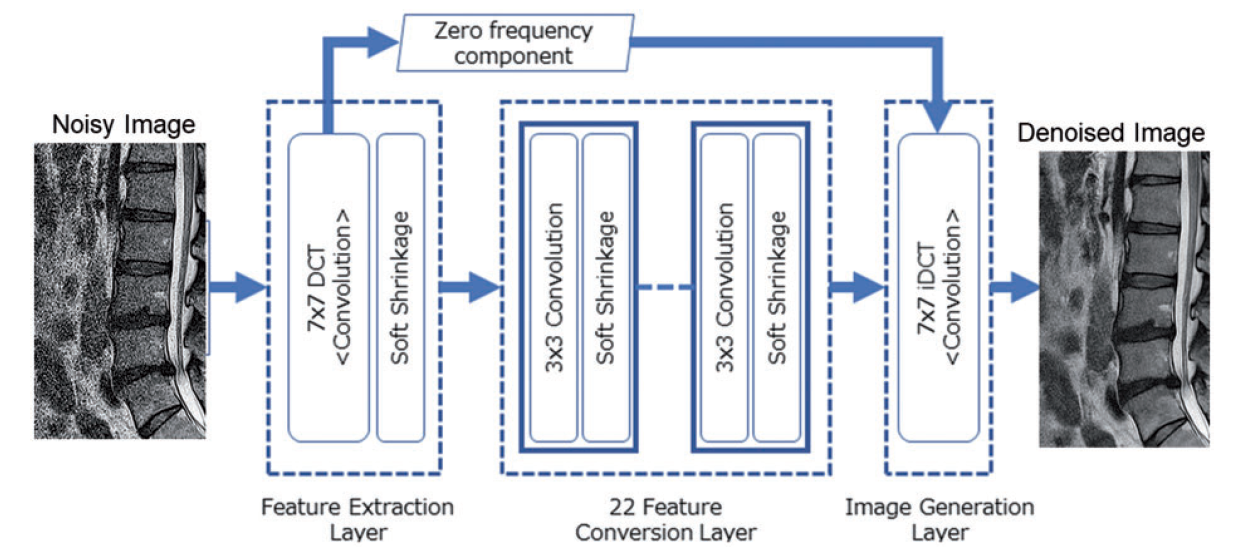
Canon's DL original AiCE method for AI-based post-processing noise reduction. A 7x7 discrete cosine transform (DCT) in the Feature Extraction Layer is first used to separate the zero frequency component from 48 others with higher frequencies. These undergo repeated 3x3 convolutions and soft shrinkages. The AI-denoised high-pass components are then upsampled and expanded in the Image Generation Layer using inverse convolution, being recombined with the bypassed
zero-frequency component.
DL-based Image Acquisition and Reconstruction
By comparison to post-processing methods, reconstruction-based AI has greater potential because it can utilize raw k-space data as well as other information such as coil sensitivities and phase. AI methods may even interact dynamically with the process of image acquisition. For example, AI may be used to stop image acquisition once a certain threshold has been reached or to recognize corrupted data samples and repeat them.
Although generic techniques have been developed, for the most part AI-based reconstruction methods are vendor-dependent and highly proprietary. Examples include Siemens' Deep Resolve, GE's Air™Recon DL, and Philips' SmartSpeed. Based on sales literature and discussions with industry insiders, these vendor-based techniques are all most useful in undersampled parallel imaging applications. Deep learning methodology may begin at the level of signals from individual coils before they are combined using information about individual coil sensitivities and noise maps. DL may also be applied to fully combined complex data during the k-space reconstruction process. The desired signal-to-noise level from this DL-enhanced hybrid construction can be adjusted by a radiologist-selectable tuning parameter (low/medium/high).
Advanced Discussion (show/hide)»
No supplementary material yet. Check back soon!
References
Clifford B, Conklin J, Huang SY, et al. An artificial intelligence-accelerated 2-minute multi-shot echo planar imaging protocol for comprehensive high-quality clinical brain imaging. Magn Reson Med 2022; 87:2453–2463. [DOI LINK]
Hammernik K, Schlemper J, Qin C, et al. Systematic evaluation of iterative deep neural networks for fast parallel MRI reconstruction with sensitivity-weighted coil combination. Magn Reson Med 2021;
86:1859–1872. [DOI LINK]
Kashiwagi N, Tanaka H, Yamashita Y, et al. Applicability of deep learning-based reconstruction trained by brain and knee 3T MRI to lumbar 1.5T MRI. Acta Radiologica Open 2021; 10:1-10. [DOI LINK]
Knoll F, Hammernik K, Zhang C, et al. Deep-learning methods for parallel magnetic resonance image reconstruction: a survey of the current approaches, trends, and issues. IEEE Signal Process Mag 2020; 37:128-140. [DOI LINK]
Lebel RM. Performance characterization of novel deep learning-based MR image reconstruction pipeline. arXiv:2008.06559 [eess.IV] 2020. [DOI LINK] (description of GE's AIR Recon DL)
Lin DJ, Johnson PM, Knoll F, Lui YW. Artificial intelligence for MR image reconstruction: an overview for clinicians. J Magn Reson Imaging 2021; 53:1015-28. [DOI LINK]
Montalt-Tordera J, Muthurangu V, Hauptmann A, Steeden JA. Machine learning in magnetic resonance imaging: Image reconstruction. Physica Medica 2021; 83:79-87. [DOI LINK]
Philips. SmartSpeed Science Brief. Koninklijke Philips NV, 2021. (sales brochure)
Rudie JD, Gleason T, Barkovich MJ, et al. Clinical assessment of deep learning-based super-resolution for 3D volumetric brain MRI. Radiology: Artificial Intelligence 2022; 4(2):e3210059. [DOI LINK]
Souza R, Bento M, Nogovitsyn N, et al. Dual-domain cascade of U-nets for multi-channel magnetic resonance image reconstruction. Magn Reson Imaging 2020; 71:140-153. [DOI LINK]
Zhu B, Liu JZ, Cauley SF, et al. Image reconstruction by domain-transform manifold learning. Nature 2018; 555:487–492. [DOI LINK] (description of the vendor-independent AUTOMAP method).
Zhu G, Jiang B, Tong B et al. Applications of deep learning to neuro-imaging techniques. Front Neural 2019;10:869. [DOI LINK]
Clifford B, Conklin J, Huang SY, et al. An artificial intelligence-accelerated 2-minute multi-shot echo planar imaging protocol for comprehensive high-quality clinical brain imaging. Magn Reson Med 2022; 87:2453–2463. [DOI LINK]
Hammernik K, Schlemper J, Qin C, et al. Systematic evaluation of iterative deep neural networks for fast parallel MRI reconstruction with sensitivity-weighted coil combination. Magn Reson Med 2021;
86:1859–1872. [DOI LINK]
Kashiwagi N, Tanaka H, Yamashita Y, et al. Applicability of deep learning-based reconstruction trained by brain and knee 3T MRI to lumbar 1.5T MRI. Acta Radiologica Open 2021; 10:1-10. [DOI LINK]
Knoll F, Hammernik K, Zhang C, et al. Deep-learning methods for parallel magnetic resonance image reconstruction: a survey of the current approaches, trends, and issues. IEEE Signal Process Mag 2020; 37:128-140. [DOI LINK]
Lebel RM. Performance characterization of novel deep learning-based MR image reconstruction pipeline. arXiv:2008.06559 [eess.IV] 2020. [DOI LINK] (description of GE's AIR Recon DL)
Lin DJ, Johnson PM, Knoll F, Lui YW. Artificial intelligence for MR image reconstruction: an overview for clinicians. J Magn Reson Imaging 2021; 53:1015-28. [DOI LINK]
Montalt-Tordera J, Muthurangu V, Hauptmann A, Steeden JA. Machine learning in magnetic resonance imaging: Image reconstruction. Physica Medica 2021; 83:79-87. [DOI LINK]
Philips. SmartSpeed Science Brief. Koninklijke Philips NV, 2021. (sales brochure)
Rudie JD, Gleason T, Barkovich MJ, et al. Clinical assessment of deep learning-based super-resolution for 3D volumetric brain MRI. Radiology: Artificial Intelligence 2022; 4(2):e3210059. [DOI LINK]
Souza R, Bento M, Nogovitsyn N, et al. Dual-domain cascade of U-nets for multi-channel magnetic resonance image reconstruction. Magn Reson Imaging 2020; 71:140-153. [DOI LINK]
Zhu B, Liu JZ, Cauley SF, et al. Image reconstruction by domain-transform manifold learning. Nature 2018; 555:487–492. [DOI LINK] (description of the vendor-independent AUTOMAP method).
Zhu G, Jiang B, Tong B et al. Applications of deep learning to neuro-imaging techniques. Front Neural 2019;10:869. [DOI LINK]
Related Questions
What causes magnetism?
What causes magnetism?